DQ's
docx
keyboard_arrow_up
School
Grand Canyon University *
*We aren’t endorsed by this school
Course
504
Subject
Business
Date
May 31, 2024
Type
docx
Pages
7
Uploaded by UltraTitanium11798
Discuss the strategic importance of forecasting at your organization (or in one with which you are familiar). Provide two examples of ways that forecasting could improve organizational processes or strategic decisions. Support your rationale with evidence from the readings or external research.
Forecasting, a process of predicting future events based on historical data, plays a pivotal role in various domains, including economics, weather forecasting, and business strategy (Anderson et al., 2016). By enabling individuals, businesses, and governments to anticipate future scenarios, forecasting helps in making informed decisions. This proactive approach not only minimizes uncertainty but also enhances resource and risk management, leading to improved strategic planning. Consequently, it enhances operational effectiveness and competitive advantage.
Forecasting holds strategic significance for institutions like Grand Canyon University (GCU), facilitating effective resource management and informed decision-making. In
my department as a University Admissions Counselor, a prime example of this would be enrollment planning. Accurate enrollment forecasts enable GCU to align its
staffing, classroom availability, and course offerings with student demand, optimizing operational costs and enhancing student satisfaction. For instance, a study in the 'Journal of Higher Education Management' reveals that institutions using advanced forecasting models achieve more stable operations and budget management (Smith & Doe, 2018). Another crucial area is financial forecasting, which aids in predicting cash flows and assessing economic conditions to support strategic initiatives and capital projects. This type of forecasting is particularly vital for maintaining financial health and supporting sustainable growth, as evidenced by
research (Ehrenberg, 2000). This underscores the role of proactive financial planning in managing economic uncertainties. Thus, by implementing robust forecasting practices, GCU can ensure it not only meets its current academic and operational goals but also secures its long-term strategic objectives. In short, being at the university, I have seen so many changes concerning enrollment planning based on forecasting. We continuously have to work on a pivot to move with the trends of culture and how we will offer the best options for our students. References:
Anderson, D. R., Sweeney, D. J., Williams, T. A., Camm, J. D., Cochran, J. L., Fry, M. J., & Ohlmann, J. W. (2016).
Quantitative methods for business with CengageNOW
(13th ed.). Boston, MA: Cengage Learning. ISBN-13: 9781305799257
Ehrenberg, R. G. (2000, April 1). Financial Forecasts for the Next Decade.
Cornel University Library
.
https://ecommons.cornell.edu/server/api/core/bitstreams/a86fb461-0078-
4186-b321-341b4087e87d/content
Smith, J., & Doe, A. (2018). Strategic Enrollment Management: Forecasting Models for Higher Education.
Journal of Higher Education Management. https://issuu.com/aaua10/docs/jhem_38-3_2023_
Explain how error measures can help to optimize moving averages, weighted moving averages, and exponential smoothing methods. Error measures are vital for enhancing the performance of time series forecasting techniques like moving averages, weighted moving averages, and exponential smoothing. These metrics gauge the precision of forecasts by comparing the forecasted outcomes to actual observations
(Anderson, et. al., 2016)
. Key error measures include Mean Absolute Error (MAE), which shows the average absolute disparity between predicted and real values; Mean Squared Error (MSE), which highlights larger discrepancies by squaring the differences; and Root Mean Squared Error (RMSE), which assesses the average size of errors in a scale-sensitive manner. Through these error statistics, forecasters can gauge the effectiveness of a model, which is crucial for spotting overfitting or underfitting and confirming model assumptions
(Anderson, et. al., 2016)
.
Applying these error measures enables analysts to refine the settings of forecasting models for enhanced accuracy. For example, in moving averages, selecting the right
window size is critical; too small might allow too much noise, while too large could obscure important trends. In weighted moving averages, adjusting the weights assigned to more recent data points according to error measures can more accurately reflect recent shifts
(Anderson, et. al., 2016)
. Similarly, in exponential smoothing, tuning the smoothing constant based on error metrics can markedly improve the model's sensitivity to trend changes
(Anderson, et. al., 2016)
. Thus, systematic testing and modification of these parameters, driven by error evaluations, help individuals sharpen their forecasts, boosting accuracy and dependability.
References:
Anderson, D. R., Sweeney, D. J., Williams, T. A., Camm, J. D., Cochran, J. L., Fry, M. J., & Ohlmann, J. W. (2016).
Quantitative methods for business with CengageNOW
(13th ed.). Boston, MA: Cengage Learning. ISBN-13: 9781305799257
Find a current example of a linear optimization model used in your industry. Describe the industry's needs, including any unique factors, how the linear optimization model was used, and the problem or challenge it addressed. Would you suggest a different model be used? Why or why not? Support your response with rationale from the assigned readings.
At Grand Canyon University, a linear optimization model is employed to enhance academic scheduling processes. The linear optimization model is a mathematical framework used to maximize or minimize a linear objective function subject to linear constraints (Anderson et al., 2016). The university's educational industry necessitates optimizing course scheduling to balance the needs of faculty availability, student course demands, and classroom resources. The primary challenge addressed by this model is to minimize conflicts in schedules, improve course availability during peak demand times, and efficiently utilize campus facilities. The linear optimization approach systematically evaluates the feasibility of
scheduling combinations to maximize student and faculty satisfaction while adhering to resource constraints such as room capacities and instructional times. However, while a linear optimization model provides a structured approach to schedule planning, it may not completely accommodate the dynamic and unpredictable elements of academic environments, such as sudden changes in course demand or faculty availability. In such cases, a different model, such as a stochastic optimization model, could be more effective. Stochastic models handle uncertainty more robustly by incorporating randomness directly into the decision-
making process, allowing for more flexible and adaptive scheduling (Anderson et al.,
2016). Implementing such models could provide GCU with a more resilient scheduling system capable of adjusting to real-time academic needs and improving overall operational efficiency. My employer, Grand Canyon University, has made many pivotal strategic choices, including the growth of its online programs and significant upgrades to campus facilities. Optimization models could have significantly enhanced these initiatives. In
expanding online education, such models might have identified the ideal combination of courses to optimize enrollment and profit while reducing operational expenses, ensuring efficient resource allocation that aligns with student needs and
Your preview ends here
Eager to read complete document? Join bartleby learn and gain access to the full version
- Access to all documents
- Unlimited textbook solutions
- 24/7 expert homework help
market trends. This strategy mirrors the principles found in our current readings, which focus on maximizing outcomes within set constraints. Additionally, in terms of
campus development, optimization models could evaluate the investment returns from various infrastructure projects to strategically fund those that most effectively and cost-effectively boost student satisfaction and retention. Moreover, predictive analytics and modeling are increasingly important in higher education administration for fostering growth and enhancing educational results.
Additionally, leveraging optimization models in strategic choices, such as those executed by GCU, not only boosts efficiency but also promotes innovation and flexibility. Utilizing optimization techniques to shape the extent and orientation of its
online educational programs allows GCU to adjust its offerings responsively to shifting market demands and technological changes. These models are also capable
of simulating different scenarios to predict and prepare for potential future obstacles, facilitating timely and effective adaptations. As detailed in an article from
Villanova University it underscores the importance of optimization and simulation in enhancing decision-making processes within dynamic settings, equipping universities with a powerful tool to make informed, data-driven choices essential for sustaining competitive advantage and educational quality (N.A., 2018). References:
How Predictive Analytics is Transforming Higher Education. (2024, March 5).
Villanova University
.
https://www.villanovau.com/articles/bi/predictive-analytics-
higher-education/#:~:text=Predictive%20analytics%20helps%20colleges
%20anticipate,aid%20award%20offered%20to%20them.
Describe workforce scheduling, blending, and logistics problems facing your current organization or industry. What is being optimized in each of your examples and why? Which linear optimization techniques could be
applied to the examples you identified? Support your response with rationale from the readings.
In another aspect of my career within the life insurance sector, workforce scheduling is crucial. This task entails determining the optimal staffing levels required at different times to efficiently manage various activities such as customer service, claims processing, and policy underwriting. The main objective here is to balance the availability of staff with service demands to ensure customer satisfaction and manage labor costs effectively. For example, it is vital to have a sufficient number of brokers during peak hours to address inquiries promptly, thereby avoiding excessive wait times. This is essential for maintaining customer satisfaction and operational efficiency. Optimizing workforce scheduling aims to minimize discrepancies between workforce capacity and service requirements,
thereby reducing the risks associated with overstaffing and understaffing (Anderson
et al., 2016). These risks can lead to higher operational costs or compromised customer satisfaction.
Additionally, in the life insurance industry, blending and logistics challenges may involve optimizing how different tasks are distributed among departments like claims processing, underwriting, and policy administration to boost efficiency. As a broker, blending could involve finding the right balance between automated processes and human involvement, or integrating various skill sets and job roles to efficiently meet processing demands. For instance, leveraging Ethos' straightforward insurance application system to automate the application process, complemented by follow-up calls to clarify any questions regarding the quotes, exemplifies this strategy
Logistics challenges in the life insurance industry often involve the efficient management of the flow of information and documents to ensure that applications and claims are processed in a timely manner (Anderson et al., 2016). Techniques such as linear programming can be applied to address these challenges effectively. For instance, linear programming can be used to calculate the ideal staffing levels in
various departments by taking into account predicted workloads and available hours, which aids in reducing operational costs while maintaining service quality. Furthermore, integrating linear programming into scheduling and task allocation can
help identify the best distribution of tasks among employees, considering factors such as skill requirements and workload limits. These methods methodically examine potential solutions, ensuring that resource distribution is not only practical but also optimized for cost, efficiency, and overall service excellence. (Anderson et al., 2016).
Reference:
Anderson, D. R., Sweeney, D., Williams, T. A., Camm, J. D., Cochran, J. J., & Fry Michael J. (2016). Quantitative methods for business. Cengage Learning. Models that pertain to the distribution of a resource within supply chains are often referred to as networks. Distribution among these networks is key to the success of a business while also keeping cost production at a minimum. Discuss at least three ways that linear optimization models can be applied to both physical as well as abstract network problems to increase efficiency in supply chain management.
Linear optimization models are crucial in improving supply chain management through efficient resource distribution and cost minimization, particularly in network
distribution contexts. The primary use of these models is to enhance transportation and logistics efficiency. Supply chains are modeled as networks with nodes representing sources (like factories) and destinations (such as warehouses), and
edges indicating the routes between them. Linear optimization techniques are utilized to identify the most economical shipping pathways. This optimization is generally achieved through a linear programming approach known as the transportation problem, which aims to reduce the overall transportation cost while adhering to each node's supply and demand requirements (Anderson et al., 2016). Additionally, these models can handle complexities like variable transportation costs, different route capacities, and multiple transportation methods, making them flexible and practical for real-world applications.
Furthermore, linear optimization models are essential for inventory management and facility site decisions, both key abstract network challenges in supply chains (Anderson et al., 2016). In inventory management, linear programming assists in determining ideal inventory levels to save holding costs and eliminate shortages among network nodes. These models are essential for businesses that manage varied product lines and face unpredictable demand. Regarding facility location, linear optimization strategically arranges warehouses and production facilities to reduce product travel distances to the market, lowering lead times and transportation costs. Companies that successfully manage these location constraints can considerably improve the overall efficiency of their supply chains. These models provide a systematic technique for evaluating the trade-offs and interactions between various operational elements, resulting in more effective resource utilization. Reference:
Anderson, D. R., Sweeney, D., Williams, T. A., Camm, J. D., Cochran, J. J., & Fry Michael J. (2016). Quantitative methods for business. Cengage Learning.
Solving shortest-route problems enables a business organization to use their resources more efficiently and minimize expenditures, thereby increasing overall production and profit. Consider the business or industry in which you work. What is a process that could be improved by the application of a shortest route linear programming model? How would this model improve production, profit, and/or efficiency?
Grand Canyon University could significantly enhance its campus transportation and logistics by applying a shortest-route linear programming model. This approach would improve how supplies are transported to various departments, such as cafeterias, maintenance, and educational areas. Often, the current system requires multiple delivery trips around the campus, which not only consumes considerable time but also increases fuel and labor costs. By adopting a shortest route model, the
university could substantially reduce the distances that delivery vehicles need to travel by ensuring they follow the most efficient routes (Anderson et al., 2016). This adjustment would decrease travel durations, cut down on fuel usage, reduce the
Your preview ends here
Eager to read complete document? Join bartleby learn and gain access to the full version
- Access to all documents
- Unlimited textbook solutions
- 24/7 expert homework help
wear and tear on vehicles, and might even allow for a reduction in the number of vehicles required. Implementing these logistical improvements could result in notable cost reductions and quicker delivery times, enhancing overall operational efficiency.
Moreover, implementing a shortest route model can significantly improve the planning and management of the university's shuttle services for students and faculty. By optimizing shuttle routes, the model can make shuttles more readily available and decrease waiting times, thus enhancing the overall campus experience (Anderson et al., 2016). This optimization allows students and faculty to spend less time traveling across campus and more time engaging in academic or extracurricular activities, which could boost their satisfaction and academic output. For Grand Canyon University, improved transportation services can be a key feature
in attracting prospective students and faculty who prioritize convenience and efficiency. Furthermore, the savings achieved from lowered fuel expenditures and more efficient shuttle operations could be reinvested into academic programs or facility enhancements, indirectly improving the quality of education and elevating the university's prestige. Thus, this strategic improvement in campus logistics and transportation bolsters daily operational efficiency and contributes to sustained educational and institutional growth.
References:
Anderson, D. R., Sweeney, D., Williams, T. A., Camm, J. D., Cochran, J. J., & Fry Michael J. (2016). Quantitative methods for business. Cengage Learning.
Related Documents
Recommended textbooks for you
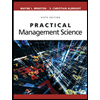
Practical Management Science
Operations Management
ISBN:9781337406659
Author:WINSTON, Wayne L.
Publisher:Cengage,
Marketing
Marketing
ISBN:9780357033791
Author:Pride, William M
Publisher:South Western Educational Publishing
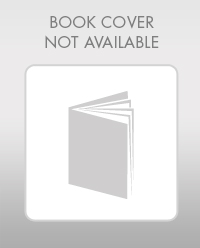
Contemporary Marketing
Marketing
ISBN:9780357033777
Author:Louis E. Boone, David L. Kurtz
Publisher:Cengage Learning
Recommended textbooks for you
- Practical Management ScienceOperations ManagementISBN:9781337406659Author:WINSTON, Wayne L.Publisher:Cengage,MarketingMarketingISBN:9780357033791Author:Pride, William MPublisher:South Western Educational Publishing
- Contemporary MarketingMarketingISBN:9780357033777Author:Louis E. Boone, David L. KurtzPublisher:Cengage Learning
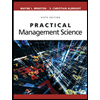
Practical Management Science
Operations Management
ISBN:9781337406659
Author:WINSTON, Wayne L.
Publisher:Cengage,
Marketing
Marketing
ISBN:9780357033791
Author:Pride, William M
Publisher:South Western Educational Publishing
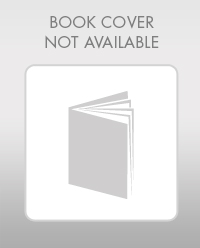
Contemporary Marketing
Marketing
ISBN:9780357033777
Author:Louis E. Boone, David L. Kurtz
Publisher:Cengage Learning